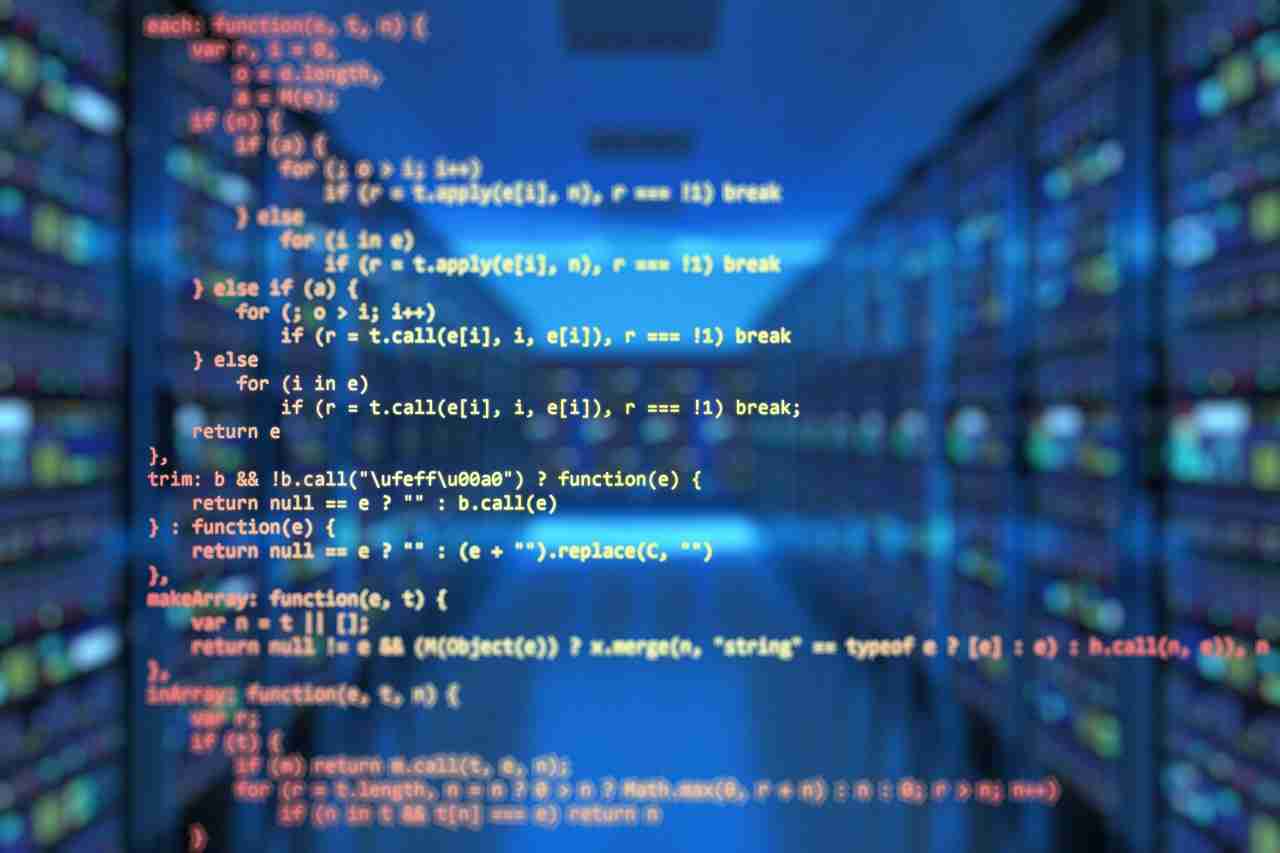
Introduction
Are you ready to dive into the exciting world of machine learning (ML)? As the demand for ML professionals continues to soar, there has never been a better time to kickstart your journey in this dynamic field. Whether you’re a seasoned data analyst looking to expand your skill set or a newcomer eager to explore the possibilities of ML, choosing the right course is essential for setting yourself up for success. In this comprehensive guide, we’ll explore actionable tips and strategies to help you select the perfect ML course and embark on your ML adventure.
Machine learning is a subfield of artificial intelligence (AI) that focuses on developing algorithms and techniques that enable computers to learn from data and improve their performance on specific tasks without being explicitly programmed. In essence, it is about creating systems that can automatically learn and improve from experience.
There are several different types of machine learning algorithms, each with its own strengths and weaknesses. Some of the most common types include:
- Supervised Learning: In supervised learning, the model is trained on a labeled dataset, where each example consists of input data along with the corresponding output label. The goal is to learn a mapping from inputs to outputs, allowing the model to make predictions on new, unseen data. Examples of supervised learning tasks include classification (predicting a categorical label) and regression (predicting a continuous value).
- Unsupervised Learning: In unsupervised learning, the model is trained on an unlabeled dataset, where there are no explicit output labels. Instead, the goal is to uncover hidden patterns and structures within the data, such as clusters or associations. Examples of unsupervised learning tasks include clustering (grouping similar data points together) and dimensionality reduction (reducing the number of features in a dataset while preserving important information).
- Semi-Supervised Learning: Semi-supervised learning is a combination of supervised and unsupervised learning, where the model is trained on a dataset that contains both labeled and unlabeled examples. The labeled examples are used to guide the learning process, while the unlabeled examples help the model to generalize better to new, unseen data.
Understanding the Landscape
Before delving into the specifics of course selection, it’s essential to understand the landscape of ML and the skills required to excel in this field. Machine learning involves developing algorithms and techniques that enable computers to learn from data and make predictions or decisions without being explicitly programmed. ML techniques are used in a wide range of applications, including image and speech recognition, natural language processing, recommendation systems, and more.
As you embark on your ML journey, it’s crucial to familiarize yourself with key concepts and methodologies in the field. Keywords like “ML course” can serve as guiding lights in your quest for resources and materials to supplement your learning. Look for online tutorials, textbooks, and case studies that provide insights into ML algorithms, tools, and best practices.
Assessing Your Skills and Goals
Before choosing an ML course, take some time to assess your current skills and goals. Are you familiar with programming languages such as Python or R? Do you have a background in mathematics and statistics? Understanding your strengths and areas for improvement will help you choose a course that aligns with your learning objectives.
For aspiring data analysts looking to transition into ML, keywords like “data analyst course” can lead to resources and courses that cover foundational concepts in data analysis and statistics. Building a strong foundation in these areas is essential for success in ML, as many ML techniques rely on statistical principles and data analysis techniques.
Choosing the Right ML Course
With a clear understanding of your skills and goals, it’s time to explore your options and choose the right ML course. Here are some factors to consider:
- Curriculum and Course Content: Look for ML courses that offer a comprehensive curriculum covering essential topics such as supervised learning, unsupervised learning, reinforcement learning, and deep learning. Keywords like “ML course” can help you narrow down your search and find courses that cover a wide range of ML techniques and algorithms.
- Instructor Expertise: Evaluate the qualifications and expertise of the course instructors. Look for courses taught by experienced ML practitioners or academics with a track record of success in the field. Instructor-led courses can provide valuable insights, guidance, and mentorship throughout your learning journey.
- Hands-on Projects and Assignments: Practical experience is essential for mastering ML techniques. Look for courses that offer hands-on projects, case studies, and assignments that allow you to apply your knowledge to real-world problems. Courses that provide opportunities for portfolio building can enhance your credibility and marketability as an ML practitioner.
- Flexibility and Accessibility: Consider your schedule and learning preferences when choosing an ML course. Look for courses that offer flexible scheduling options, self-paced learning modules, and accessible online platforms. Courses that provide lifetime access to course materials and resources allow you to revisit concepts and review content at your own pace.
- Peer Learning and Support: Learning from peers and collaborating on projects can enhance your learning experience in ML. Look for courses that foster a supportive learning community, such as online forums, discussion groups, and peer review opportunities. Courses that offer networking opportunities and connections to industry professionals can also provide valuable insights and career guidance.
Supplementary Resources and Learning Opportunities
In addition to formal ML courses, there are plenty of supplementary resources and learning opportunities to enhance your skills and knowledge. Consider exploring online tutorials, blogs, podcasts, and webinars related to ML and specific topics of interest. Keywords like “data analyst course” can lead you to resources that cover foundational concepts in data analysis and statistics, which are essential for success in ML.
Engage with online communities and forums dedicated to ML, such as Stack Overflow, Reddit, and LinkedIn groups. Participate in ML competitions, hackathons, and challenges to sharpen your skills, expand your network, and showcase your expertise.
Conclusion
Choosing the right ML course is a critical step towards achieving your goals and embarking on your ML journey. By considering factors such as curriculum content, instructor expertise, hands-on learning opportunities, flexibility, and accessibility, you can find a course that aligns with your learning objectives and sets you on the path to success.
Keywords like “data analyst course” and “ML course” can serve as guiding lights in your quest for the perfect course. Whether you’re a beginner looking to explore the basics of ML or an experienced practitioner seeking to expand your skill set, there’s a course out there to help you achieve your goals. So take the first step towards your dream career in ML, and embark on an exciting journey of discovery, learning, and growth in this dynamic and rapidly evolving field.